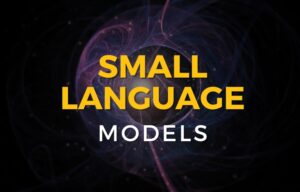
In order to stay competitive, retailers need to offer promotions and prices that are appealing to customers. But with so many variables at play, how can retailers be sure they are making the right decisions? The answer is predictive analytics.
Predictive analytics is a tool that uses data mining, machine learning, and statistical modeling to make predictions about future events. In other words, it allows retailers to see into the future and make decisions accordingly. By analyzing past data, retailers can identify patterns and trends that can be used to predict future behavior. There are a number of different methods that can be used for predictive analytics, including regression analysis, time series analysis, and machine learning. For context, regression analysis is a statistical method that is used to identify relationships between variables. while time series analysis is a statistical method that is used to examine trends over time. Machine learning, on the other hand, is a type of artificial intelligence that is used to learn from data and make predictions.
Predictive analytics can be used for a variety of purposes, but two of the most common applications are optimizing promotional strategies and setting prices. By using predictive analytics to plan promotions and pricing, retailers can make more informed decisions that ultimately result in increased sales and profits. Let’s take a closer look at how predictive analytics can be used for each of these purposes.
In order for businesses to be profitable, they need to charge prices that cover their costs while still meeting customer demand. However, prices that are too high will drive customers away, while prices that are too low will result in lost profits. Finding the perfect balance between these two extremes can be difficult, but predictive analytics can help.
By using predictive analytics to analyze past customer behavior, businesses can identify patterns in how customers respond to changes in price. For example, if a business notices that its sales increase when it lowers its prices by 10%, it may use this information to inform future pricing decisions. In this way, predictive analytics takes the guesswork out of setting prices and helps businesses optimize their pricing strategy for maximum profitability.
Another way retailers leverage predictive analytics is at the customer level. For example, if a retailer knows that a particular group of customers is willing to pay more for a product than other groups of customers, they may adjust prices accordingly in order capture additional revenue from those customers. Additionally, by understanding how changes in prices impact demand across different segments of customers, retailers can also target specific groups of customers with specific pricing structures designed specifically for them. The goal is ultimately the same as with promotions––to use predictive analytics to increase sales and profits by understanding how customers react to pricing changes. Furthermore, predictive analytics can also help companies improve customer satisfaction by ensuring that prices are fair and competitive.
Promotions are a key part of the retail landscape. They are often used to drive traffic and sales during slow periods or to clear out seasonal merchandise. However, planning and executing promotions can be a time-consuming and costly endeavor. Predictive analytics can help retailers streamline the promotion process by identifying which products are most likely to sell and when customer demand is likely to peak. This information can then be used to tailor promotional strategies accordingly. By analyzing past customer purchase data, retailers can identify patterns and trends that can be used to predict future behavior. This information can then be used to segment customers and target them with promotional offers that are more likely to convert to a sale. Not only does this result in higher response rates and more sales, but it also helps to improve customer loyalty and retention.
For example, if a retailer knows that a certain type of customer is more likely to respond positively to a discount promotion, they can tailor their campaign specifically to target that group of customers. Additionally, by understanding how customers responded to past promotions, retailers can make adjustments to future campaigns to further improve results. Ultimately, the goal is to use predictive analytics to create promotional campaigns that are customized specifically for each type of customer in order to maximize the number of customers who make a purchase.
This also works at a product level. For example, predictive analytics can identify patterns of products that are regularly purchased together. This may signal to a retailer to consider bundling these products in a promotion. Predictive analytics can give retailers a deeper understanding of their customers and buying habits to make informed decisions about promotional strategy.
Predictive analytics can be a huge help when it comes to managing inventory. By analyzing past data and trends, retailers can get a better idea of which products are selling well and which ones aren’t moving at all. This information can then be used to make more informed decisions about what to stock in the future. For example, if a retailer sees that a particular type of product is selling very well during the winter months but not so much during the summer, they may decide to increase their order quantity for that item during the winter and decrease it during the summer. This kind of strategic planning can help retailers avoid costly inventory mistakes.
Retaining existing customers costs less than acquiring new ones. Predictive analytics can help identify unhappy customers before they churn and improve retention by predicting who will leave next. It’s a way to catch unengaged customers before they completely abandon your brand. Companies can analyze this information and take the necessary actions to improve customer satisfaction and ensure their revenues remain unaffected.
Believe it or not, predictive analytics can even be used to inform store layout and design decisions. By analyzing customer traffic patterns and purchase data, retailers can identify which areas of their store are being used most frequently and which ones could be redesigned to better meet customer needs. For example, if a retailer sees that customers are spending a lot of time in the shoe department but not so much in the handbag department, they may decide to move the handbags closer to shoes in order to encourage cross-selling. Making thoughtful changes like this based on data can help increase sales and improve the overall shopping experience for customers.
Organizations can use predictive analytics for price and promotion optimization by building models that identify relationships between consumer behavior, product attributes, and trends. These models allow organizations to make real-time decisions about pricing and promotions based on data rather than intuition or guesses. As a result, companies can optimize prices and rises to meet customer demand without losing margin or sacrificing revenue.
Contact us today if you’re interested in learning more about how we can help you optimize your retail operations using predictive analytics.
WEBINAR