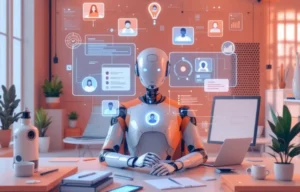
Artificial General Intelligence (AGI) represents the concept of machines reaching human-level intelligence, enabling them to understand, learn, and apply knowledge autonomously across diverse tasks. While AI has made incredible strides in specific domains—such as language processing and image recognition—AGI poses a unique challenge because it demands a broader, adaptable intelligence closer to human cognition. In this blog post, we’ll explore the framework and components that researchers and technologists are developing to make AGI a reality. For readers looking to understand AGI’s foundational concepts and current advancements, [Quantilus’s AI blog series]provides a great starting point.
To reach AGI, it’s essential to understand its difference from narrow AI (ANI) and the path needed to achieve it. While ANI is designed to perform specific tasks, AGI aims to mimic human intelligence broadly. This means AGI must excel in areas like reasoning, planning, and creativity—qualities currently beyond traditional machine learning models.
Key frameworks and methodologies for developing AGI include hybrid AI models that blend symbolic reasoning with neural networks. For example, combining reinforcement learning and cognitive architectures is seen as one potential pathway. To dive deeper into this approach, check out [OpenAI’s exploration of reinforcement learning and neural networks]. OpenAI continues to push boundaries in AGI research by focusing on how reinforcement learning can enable machines to make decisions based on previous outcomes, emulating human trial and error.
A foundational step toward AGI is building cognitive architectures that mimic human brain function. These architectures simulate neural processes and attempt to recreate complex decision-making pathways found in human cognition. Neuromorphic computing—a field that designs computer systems inspired by the human brain—is an emerging approach. Chips from companies like IBM and Intel simulate the brain’s structure, allowing machines to operate faster and more efficiently in tasks requiring pattern recognition.
For those interested in the latest in neuromorphic engineering, Intel’s Loihi research project explores how brain-like processors can facilitate AGI. By simulating how the brain’s neurons fire, neuromorphic chips provide machines with more advanced sensory processing capabilities, which is a step towards replicating human-like awareness.
Ethics and safety remain primary concerns for AGI researchers, as the implications of AGI could profoundly impact society. Ensuring AGI systems align with ethical guidelines is critical to prevent unintended harm. Organizations like OpenAI and the [Partnership on AI]work on creating frameworks for AI transparency, accountability, and alignment with human values.
The concept of “explainable AI” (XAI) plays a significant role in AGI ethics. Explainable AI focuses on making machine decisions transparent and understandable for human stakeholders. Readers can learn more about the latest developments in ethical AI by visiting AI research blog, where Google AI frequently discusses how to make AI systems safer and more transparent.
As AGI develops, so too does the importance of security. Cybersecurity experts emphasize the risks that AGI could pose in terms of data privacy and malicious use. Currently, AI-based defenses are enhancing cybersecurity through predictive analysis and anomaly detection. This trend is expected to grow in AGI, as these systems will need robust security protocols to prevent breaches and attacks.
The [Cybersecurity and Infrastructure Security Agency (CISA) ] has extensive resources on how AI impacts cybersecurity, including practical guides on safeguarding AI systems against threats. For anyone interested in understanding the challenges AGI security presents, CISA’s resources are highly recommended.
Data diversity and volume are critical in training an AGI system. Unlike narrow AI, AGI needs to generalize knowledge across domains, which requires a comprehensive dataset encompassing diverse scenarios and problems. This is where synthetic data comes in, allowing for data generation at scale. Google and NVIDIA, for example, are leveraging synthetic data to train their models, addressing gaps in real-world data availability.
To explore the transformative role of synthetic data, visit NVIDIA’s [AI research blog]. Their blog dives into how synthetic data generation supports various AI applications, from gaming to healthcare, demonstrating how diversified data helps build robust AI systems.
Creating AGI will involve multiple scientific fields and innovations. Cognitive architectures, neuromorphic computing, ethical frameworks, security protocols, and comprehensive datasets will all play crucial roles in advancing AGI. The journey to AGI is intricate and multi-faceted, but the framework discussed here outlines the promising paths and technologies that can bring us closer to achieving it.
WEBINAR