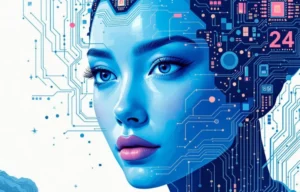
As AI continues to reshape industries, recent research breakthroughs at MIT signal a transformative leap in robot training. This innovation leverages advanced generative AI and machine learning models to speed up how robots learn, making automation more efficient, accurate, and scalable. MIT’s work integrates several of the AI trends dominating conversations today, from generative AI in business and cybersecurity to the growing importance of ethics and prompt engineering.
Â
Let’s examine the significance of MIT’s research and explore how it relates to the latest trends in AI.
Â
MIT researchers have introduced a framework that enables robots to learn complex tasks faster by integrating simulation environments with real-world feedback loops. This hybrid training approach relies on retrieval-augmented generation (RAG) and generative AI models—making robots smarter by allowing them to access external data sources in real time. This breakthrough holds tremendous promise for industries ranging from logistics and healthcare to manufacturing.
Â
To understand how retrieval-augmented generation works and its impact on automation, check out TechRepublic.
Â
This innovation also aligns with the growing use of digital twins, which simulate physical systems to optimize performance and predict outcomes. With digital twins, a robot’s virtual counterpart can be trained in a controlled simulation, while real-world data continuously refines its learning process Exploding Topics.
Â
A crucial element of MIT’s research is its adherence to ethical AI frameworks. Robotics and AI deployments face risks like inaccuracy, bias, and intellectual property issues. To address these concerns, MIT’s initiative aligns with emerging AI governance standards such as the European Union’s AI Act.
Â
Transparent practices, such as **watermarking content generated by ​Coursera.
Â
AI-driven robots also raise cybersecurity concerns, particularly regarding data privacy and model vulnerabilities. McKinsey & Company ensures compliance with the latest security protocols, following McKinsey’s recommendations for responsible AI governance.
Â
MIT’s breakthrough underscores the increasing importance of prompt engineering—a key skill that optimizes how robots and AI systems interpret and act on human commands. Just as developers use tools like GitHub Copilot or Tabnine to automate code suggestions, engineer​ robot behavior through precisely structured prompts.
Â
This shift highlights a broader trend where prompt engineering becomes essential not just for developers, but also for managers and non-technical users who interact with AI systems regularly.
Â
MIT’s hybrid training model leverages generative AI models, enabling robots to quickly generate scenarios and solutions. This aligns with the broader trend of businesses integrating generative AI into daily operations. Whether it’s supply chains or enhancing HR management, these tools offer faster problem-solving and reduced costs.
Â
However, generative AI also presents risks such as inaccurate outpu​ al property challenges. Companies, including MIT’s partners, are adopting watermarking and metadata techniques to maintain transparency, similar to Adobe’s practices in the creative industry.
Â
With advanced AI models powering robotic systems. Robots operating on interconnected platforms are vulnerable to data breaches, requiring robust security measures. MIT’s training framework integrates real-time analytics to detect anomalies early, ensuring safe operation.
Â
MIT’s research marks a pivotal moment in robot training, accelerating their ability to perform complex tasks autonomously. This breakthrough reflects many of today’s top AI trends—from retrieval-augmented generation to generative AI in business—while tackling regulatory, ethical, and security challenges head-on. The synergy between AI-enhanced robotics and digital twins paves the way for smarter, safer automation across industries.
Â
As these innovations unfold, staying the Quantilus blog for more insights and expert commentary on emerging technologies.
WEBINAR